Straight out of science fiction, coming soon to clinics around the world is a new breed of healthcare providers – powered by artificial intelligence. From diagnostics to records management and even clinical trials, data-driven technologies enhance accuracy and access, and reduce the strain on the human workforce.
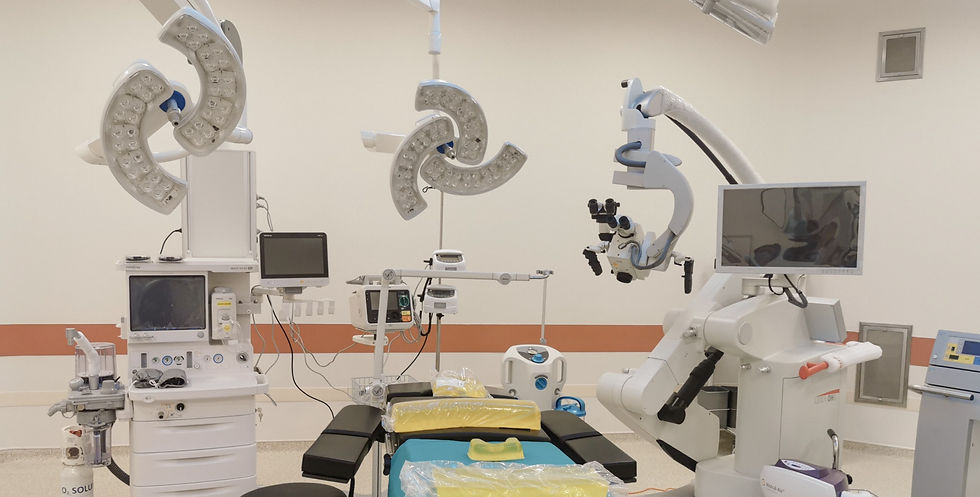
The reach of artificial intelligence (AI) in modern life is broad and deep, propelled by the vast data training sets now available to refine technologies. Access to data is a key precondition for machine learning, which improves through repetition and continued testing of assumptions and probabilities.
From gaming to agricultural applications, AI use is increasingly common. In the health sector the introduction of automated processes requires even more vigorous testing and an expert workforce to ensure that AI will do what all doctors are implored to do under the Hippocratic Oath—namely, no harm.
According to the World Health Organization’s inaugural 2021 report on AI in healthcare, Ethics and governance of artificial intelligence for health: “For AI to have a beneficial impact on public health and medicine, ethical considerations must be placed at the centre of the design, development, and deployment of AI technologies for health.”
AI presents a huge opportunity for healthcare systems. Machine learning applications can be deployed to aid diagnostics, clinical decision-making and workflow optimisation, reducing the strain on the human workforce.
Health records management and interpretation have been an obvious starting point and source for machine learning, but the growing intelligence of these systems can expand into patient care, enabling complex treatments within smaller budgets.
Delivering at national scale
AI is getting increasingly sophisticated at performing human tasks in healthcare settings—often more efficiently, faster and at lower cost. So significant is the potential of AI in healthcare that the global market size is expected to reach US$187.95 billion by 2030—up from US$11.06 billion in 2021.
North America is the world’s largest market, while Asia-Pacific is the fastest growing region. In Japan, the government has funded a major national initiative that will use AI to increase the efficiency and quality of healthcare delivery. Imaging and pathology tests, automated record-keeping and assistance robots are some of the areas in which AI intervention is planned.
SGInnovate, a government-backed Singaporean company specialising in deep tech, aims to advance the development and adoption of AI in mainstream healthcare settings to improve diagnostics, treatment and clinical outcomes.
The covid-19 pandemic has been a significant catalyst for the development, says CEO Dr Lim Jui. “We needed to minimise contact and hospitals were overloaded,” Jui says. “There had to be a better way to perform pathology, for example, or ultra-stenography, to relieve the load. The pandemic spurred companies on to develop these technologies.”
He says SGInnovate looks after a portfolio of start-ups that focus on AI applications specific to healthcare, including echocardiography, testing of pathological specimens and predictive healthcare. Also within its portfolio are start-ups developing technological tools such as a robotic biopsy device, which analyses a variety of imaging sources, and an AI assistant that will assist medical professionals with protocols for tissue sampling and other processes.
As with AI development in other industries, sufficient data sets that enable machine learning are vital to the success of AI applications in healthcare. If cancer is the focus, for example, the system must be provided with both cancerous and non-cancerous specimens, and given clear rules for processing pathology images.
“That initial training set is important,” says Jui. “It must help the machine derive its operating boundaries and operating assumptions, and provide a sufficiently diverse range of pathologies in different stages of the natural history of a disease, for the machine to learn properly. That’s still the biggest challenge.”
Given that repeatable, standardised procedures help machines improve their understanding of underlying indications, Jui sees mental health as one of the big emerging areas in AI-assisted healthcare, because it is a language-derived discipline that can leverage the language capabilities machine learning has acquired in recent years.
Indeed, research shows users of mental health chatbots like Woebot and Tess experience significantly reduced symptoms of depression and anxiety. Smartphone apps like these can provide care to large, disparate populations who may not otherwise have access to mental health support. One 2021 study involving Argentinian students, published in JMIR Formative Research, reported “promising evidence for the usability and acceptability of Tess in the Argentinian population”.
Cancer screening assistant
The diagnosis, treatment, management, and prevention of non-communicable diseases (NCDs) will be one of the largest frontiers for AI in healthcare in the coming years. Specifically, AI-based diagnostic tools are already being deployed to identify a range of cancers, advanced by not only large data sets to assist machine learning, but also by a wealth of existing research information, which AI is able to absorb and interpret.
The target is to screen at least 70% of women worldwide by 2030. Importantly for women in LMICs, AI-based pathology tools will bypass the current shortage of medical expertise.
According to a 2021 WHO report examining AI-based software as medical devices, evidence shows AI systems may soon be used in screening for skin cancer, lung cancer, breast cancer, cervical cancer and numerous other malignant and premalignant conditions. Cervical cancer screening, which is done periodically and on a large scale, can quickly scale up the efficiency of an AI application, particularly in low- and middle-income countries (LMICs).
Cervical cancer remains one of the most common and yet preventable cancers in women worldwide. Women in LMICs are disproportionally affected, accounting for an estimated 85 per cent of the 500,000 new cases and 311,000 deaths reported globally in 2018.
Given the known cause of the disease, the uniformity of the test and its high preventability, a high-quality AI diagnostic tool deployed at scale can aid the WHO’s global strategy to eliminate cervical cancer. The target is to screen at least 70% of women worldwide by 2030. Importantly for women in LMICs, AI-based pathology tools will bypass the current shortage of medical expertise.
Johan Lundin, a professor in the Department of Global Public Health at Karolinska Institutet, says AI can save hours in analysing cell samples. In a recent study at a rural clinic in Kenya, pap test results from 740 women were collected, digitised and analysed using a deep learning algorithm. The AI was 96 per cent accurate.
“The combination of AI and a human expert can enable analysis of five to 10 times more samples per day than what is currently possible and with a greatly improved reproductibility,” says Lundin, a co-author of the study. “The AI acts as a tireless virtual assistant that scans digitised pap smears for abnormal cells 24 hours per day, 7 days a week.”
The human expert verifying the AI findings need not be on-site, since the digitised cervical pap smears can be uploaded to a cloud environment and AI results evaluated remotely, explains Associate Professor Nina Linder, another co-author. “This is a significant step towards more equal and sustainable access to high-quality diagnostics in resource-limited settings.”
Clinical trials reimagined
AI is also transforming the way we conduct scientific research. The randomised clinical trial—which is a must before commercialising any new drug, therapy, or medical knowledge—entails preclinical testing, participant recruitment and several phases of randomised testing, which can take years, with scant guarantee of success.
For the last three years, Associate Professor Sunil Gupta and the team at the Applied Artificial Intelligence Institute at Deakin University have been working on adaptive trials—an improvised version of the randomised clinical trial where AI is used to look at previous impacts based on existing data. This minimises the harm to participants and increases the probability of good outcomes.
“Randomised clinical trials have been at the core of trialling any drugs, treatments and interventions for healthcare,” says Gupta. “Traditional fixed-allocation trials are largely inefficient and costly, which hinders the discovery of new knowledge. Another issue with randomised clinical trials is that they cannot be conducted using existing data—they always need to go live.”
The key challenge is to familiarise the healthcare community with the power of AI and develop a method of reporting data that protects patient confidentiality so that stakeholders feel comfortable using these methodologies.
AI-based methods allow for personalised solutions, says Gupta. “AI methods are capable of working with pre-existing offline data, in scenarios where recruiting certain types of subjects is not possible or trialling the interventions is risky.
“AI methods can predict the effects of an intervention for a target population based on its learning from data from a related population.”
An example is “offline” data collected at a hospital or clinic, he explains. While this data is not collected within a randomised clinical trial framework, AI methods can harness the data to achieve similar discoveries. Likewise, AI methods can help generalise the outcome of a trial—the efficacy of a treatment, for example—in one country to new populations, which saves cost and widens the reach of the trial.
The key challenge is to familiarise the healthcare community with the power of AI and develop a method of reporting data that protects patient confidentiality so that stakeholders feel comfortable using these methodologies.
Gupta and his colleagues now have funding to develop a platform on which they can run adaptive trials. An ongoing trial is on the efficacy of mental health interventions for university students for indications such as depression, anxiety, and stress. Instead of testing a fixed set of therapies on a fixed number of people, AI is used to ensure that a maximum number of participants are allocated the therapy that works best for each of them, Gupta says.
Importantly, the need for a medical workforce doesn’t evaporate with the entry of AI. “We still need a medical workforce,” Gupta says. AI will help determine which intervention would work best for each participant, he says.
“Two things then happen: this person has an overall good experience and benefits from the intervention; and, secondly, we, as scientists, find out quickly which interventions are working and which are not, in the smallest length of the trial. AI is the underlying technology which is ensuring this.”
The FDA approved adaptive trials two years ago, and a gradual understanding of this research method mirrors a wider acceptance of the role—and, indeed, potential—of AI in healthcare. “Since then, people have felt a bit more comfortable about the involvement of AI in a sector as sensitive as healthcare,” says Gupta.
Case study: Closing the sight gap
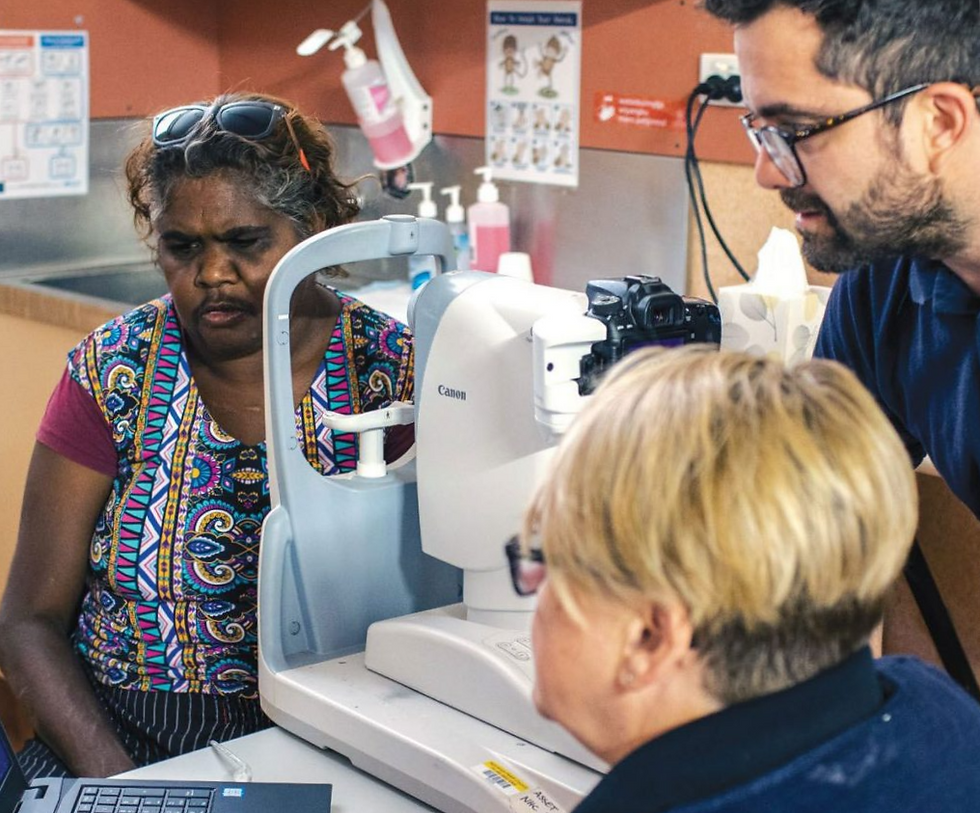
Indigenous Australians are almost four times more likely to develop diabetes than non-Indigenous Australians, and one in 10 will go on to develop vision-threatening diabetic retinopathy. This disparity is indicative of the vast health and life expectancy inequalities that persist between the two groups.
To help close the health gap, the Centre for Eye Research Australia (CERA), which is affiliated with the University of Melbourne, is currently using an AI tool to conduct eye tests in seven remote Indigenous communities in South Australia.
The AI tool’s algorithm was developed and tested over five years using more than 200,000 images of the back of the eye as a training set. It now reviews retinal images and can identify eye diseases within seconds.
The tool can be operated by a local nurse and the results are immediate. The images no longer need to be sent hundreds of kilometres away to the nearest major city for analysis by an ophthalmologist. This has important implications for early intervention, with the potential to save the sight of thousands of patients.
“Currently, people living in remote areas have to travel eight to 10 hours to see the optometrist on fixed days… and because it’s inconvenient they are unlikely to come again,” says Professor Mingguang He, head of ophthalmic epidemiology at CERA and one of the trial leads. “Then patients wait around two weeks for the report.
“With AI technology we will be able to provide point-of-care service where a report can be provided immediately after screening.”
He says the researchers aim to get the AI-based screening service subsidised by the Australian government so “people in remote and Indigenous communities can enjoy the service in the long run”.
Comments